AUTHOR(S) / АУТОР(И): Dušan Marković, Uroš Pešović, Dalibor Tomić, Vladeta Stevović
E-MAIL: dusan.markovic@kg.ac.rs
Download Full Pdf
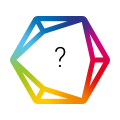
DOI: 10.46793/SBT28.093M
ABSTRACT / САЖЕТАК:
Weeds are one of the most important factors affecting agricultural production. Environmental pollution caused by the application of herbicides over the entire agricultural land surface is becoming more and more obvious. Accurately distinguishing crops from weeds by machines and achieving precise treatment of only weed species is one possibility to reduce the use of herbicides. However, precise treatment depends on the precise identification and location of weeds and cultivated plants. The aim of the work was to describe and point out the importance of deep learning models for the detection and classification of weeds, in order to enhance their application in real conditions.
KEYWORDS / КЉУЧНЕ РЕЧИ:
agriculture, image processing, artificial neural network, weed detection, weed control
REFERENCES / ЛИТЕРАТУРА:
- Assunção E., Gaspar P., Mesquita R., Simões M., Alibabaei K., Veiros A., Proença H. (2022). Real-Time Weed Control Application Using a Jetson Nano Edge Device and a Spray Mechanism. Remote Sensing 2022, 14, 4217.
- Keras, https://keras.io/, last visited: 07.02.2023.
- O’Donoghue E.R.A., Hoppe D.E., Banker R., Ebel K. Fuglie P.K. (2011). The changing organization of US farming. USDA Economic Research Service. Economic Information Bulletin. United States Department of Agriculture EIB No. 88.
- Razfar N., True J., Bassiouny R., Venkatesh V., Kashef R. (2022). Weed detection in soyabean crops using custom lightweight deep learning models. Journal of Agriculture and Food Research, 8, 100308.
- Šćepanovi M., Sinan A., Šoštarčić V., Brijačak E., Pintar A., Barić K. (2018). New methods and approaches to precise weed control. Bulletin of plant protection, 18(5), 488-499.
- Singh A., Ganapathysubramanian B., Singh A.K., Sarkar S. (2016). Machine learning for high-throughput stress phenotyping in plants. Trends Plant Sci.
- Wang A., Zhang W., Wei X. (2019). A review on weed detection using ground-based machine vision and image processing techniques. Computers and Electronics in Agriculture, 158, 226-240.
- Wu Z., Chen J., Zhao B., Kang H., Ding Y. (2021). Rewiew of weed detection methods based on computer vision. Sensors, 21, 3647.